Tutorial
Dec 19, 2024
Overcoming Transcription Challenges for Multilingual AI voice agents
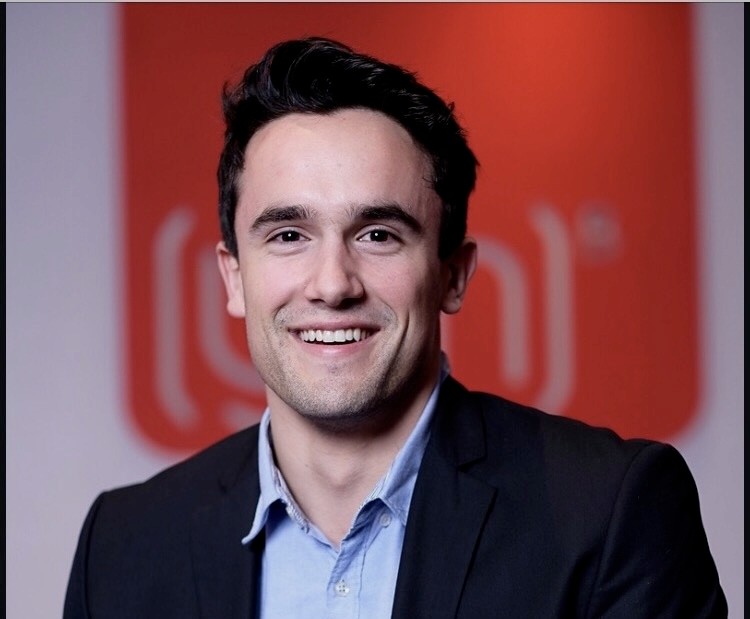
Michael Louis
CEO & Founder
The landscape of voice-based artificial intelligence is rapidly evolving, however many of our customers at Cerebrium have brought to our attention the limitations when it comes to multilingual applications. Recently, support for LLMs has improved for different languages. Cartesia, being one of the proponents for these improvements, have just released increased support for their TTS (Text-to-speech) service; with support for over 6 languages (French, Chinese, Spanish etc). Their localization features allow users to recreate an accent of a specified language, together with a gendered voice.
One area lacking in multi-lingual support seems to be STT (Speech-to-text) services, as they seem to miss the spelling or order of words. This makes it difficult to implement in production as well as, to pass transcriptions into downstream LLMs. For services that do do well here like Azure’s Speech to Text service, they either have a high latency (higher than what's acceptable for real time voice capabilities) or are too expensive to make the unit economics of voice calling work.
In this tutorial we will show you how you can create a french speaking voice agent. Specifically, we will focus on methods you can implement to lower the WER (Word Error Rate) for an STT model while still keeping latency and cost low.
You can find the final code for this tutorial in this example repository.
Reducing WER
Whisper v3 is an extremely good STT model and supports a variety of languages, however, its support of non-english languages is sub-par and is, in some cases, not production ready.
The figure below shows a performance breakdown of large-v3
and large-v2
models by language, using WERs (word error rates) or CER (character error rates, shown in Italic) evaluated on the Common Voice 15 and Fleurs datasets
To decrease this error rate, we will be using fine-tuned Whisper models from Hugging face. These perform much better than the default whisper-v3 model and seem to have much lower error rates. Some of our favourites that customers have in production are:
Before we start, all of these fine-tuned whisper models will be implemented using Faster-Whisper which uses Ctranslate2 (A fast inference engine for Transformer models). This implementation is up to 4 times faster than the traditional whisper model, with no effect on accuracy.
Tutorial
If you don’t have a Cerebrium account, you can create one by signing up here and following the documentation here to get set up
In your IDE, run the following command to create our Cerebrium starter project: cerebrium init 8-multilingual-twilio-agent
. This creates two files:
main.py - Our entrypoint file where our code lives
cerebrium.toml - A configuration file that contains all our build and environment settings Add the following pip packages near the bottom of your cerebrium.toml. This will be used in creating our deployment environment.
Above, we use the custom runtime setting from Cerebrium since we need to implement Web sockets via FastAPI in order to communicate with our Twilio phone call. Lastly, we instal all the required pip packages needed to run our application.
We need to setup a FastAPI server that will receive the call from our Twilio number and then upgrade the connection to a websocket connection - this is for the real-time two-way communication. To setup a Fast API server on Cerebrium is the same as setting up FastAPI locally. You can then add the following code to your main.py:
Don’t worry about the line from bot import main
- we will add this later. In your current directory, create a folder called ‘templates’ and inside it a file called ‘stream.xml’. We need to send back a xml response to Twilio in order to upgrade the connection to the websocket connection. Add the following code to the streams.xml file:
The stream url will be the base endpoint of your deployment once our application is deployed. It should be the same in the above, you should just fill in your project id. Before we continue with our implementation, let us setup our Twilio number.
Twilio setup
Twilio is a cloud communications platform that enables businesses to integrate messaging, voice, video, and authentication capabilities into their applications through APIs. We will use them for this demo, but you can use any alternative provider. If you don’t have an account you can sign up here - they have a generous free tier.
Once you have an account, you can navigate to the page in order to buy a number. When you buy a number please make sure its not a toll-free number - this won’t work. We then setup a webhook that hits our endpoint in order to connect to the agent.
You should then save the changes above and move on to setting up our AI Agent.
AI Agent Setup
In order to create our AI agent, we will be using the PipeCat framework that takes care of stringing together all the components and it handles some of the functionality we might need such as user interruptions, dealing with audio data etc. To setup our AI agent, let us create another file called ‘bot.py’ and add the following code:
The code above does the following:
We connect our agent to our websocket transport layer that will send/receive audio.
We setup our LLM, TTS and STT services using Faster Whisper, OpenAI and Cartesia. You can select any provider you like - Pipecat supports a wide variety.
In order to authenticate all these services, we use Secrets. Add them to your Cerebrium dashboard.
Lastly, we then put this all together as a PipelineTask which is what Pipecat runs all together. The makeup of a task is completely customisable and has support for Image and Vision use cases. You can read more here. Pipeline tasks come with a structure and parameters that make it easy to handle interruptions out the box, and we are able to swap models to our preference only changing a few lines of code.
The Daily Python SDK comes with a lot of event webhooks where you can trigger functionality based on events occurring. So we handles certain events such as a user leaving/joining a call.
In order to improve the latency of the above system, you can run parts/all of the pipeline locally. This would help you achieve roughly ~500ms end-to-end latencies. You can read more how we did this here and here.
Deploy to Cerebrium
To deploy this app to Cerebrium you can simply run the command: cerebrium deploy in your terminal.
If it deployed successfully, you should see something like this:
Remember, once you have your deployment url, you need to update your streams.xml file and redeploy. In order to test this application you can simply call your Twilio number and the agent should start responding.
Conclusion
By following this tutorial, you’ve successfully built a French-speaking voice agent capable of real-time conevrsation, leveraging Cerebrium’s infrastructure for efficient deployment and Twilio’s robust communication platform. You’ve learned how to reduce WER (Word Error Rate) for multilingual speech-to-text processing using fine-tuned Whisper models, ensuring both accuracy and low latency. Additionally, the integration of Faster Whisper and Pipecat has enabled a streamlined, scalable setup with customizable pipelines and seamless interaction.
This foundation equips you to extend the agent’s capabilities, whether by supporting additional languages, optimizing for other use cases, or further reducing latency. With Cerebrium, you now have the tools to innovate in voice-based AI applications and address the challenges of multilingual deployments.
Tag us as @cerebriumai so we can see what you build and please feel free to ask questions/send feedback to us on Slack or Discord communities